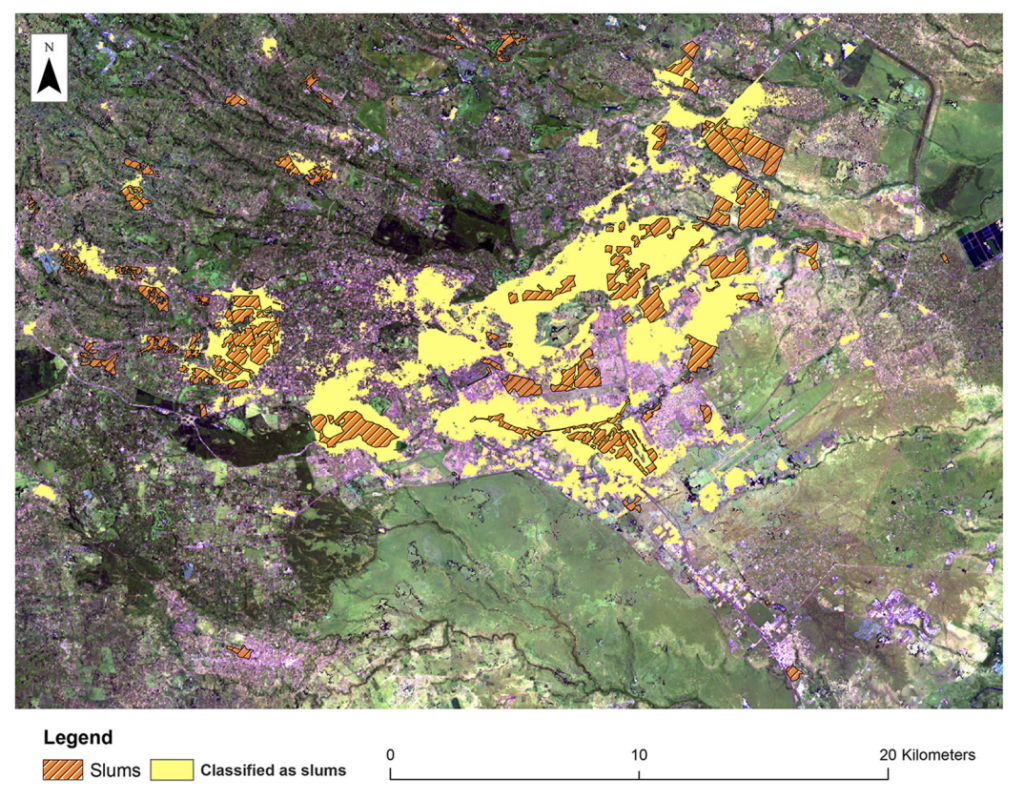
The worldwide slum population currently stands at over one billion, with substantial growth expected in the coming decades. Traditionally, slums have been mapped using information derived mainly from either physical indicators using remote sensing data, or socio-economic indicators using census data. Each data source on its own provides only a partial view of slums, an issue further compounded by data poverty in less-developed countries. To overcome such issues, this paper explores the fusion of traditional with emerging open data sources and data mining tools to identify additional indicators that can be used to detect and map the presence of slums, map their footprint, and map their evolution. Towards this goal, we develop an indicator database for slums using open sources of physical and socio-economic data that can be used to characterize slum settlements. Using this database, we then leverage data mining techniques to identify the most suitable combination of these indicators for mapping slums. Using three cities in Kenya as test cases, results show that the fusion of these data can improve the mapping accuracy of slums. These results suggest that the proposed approach can provide a viable solution to the emerging challenge of monitoring the growth of slums.