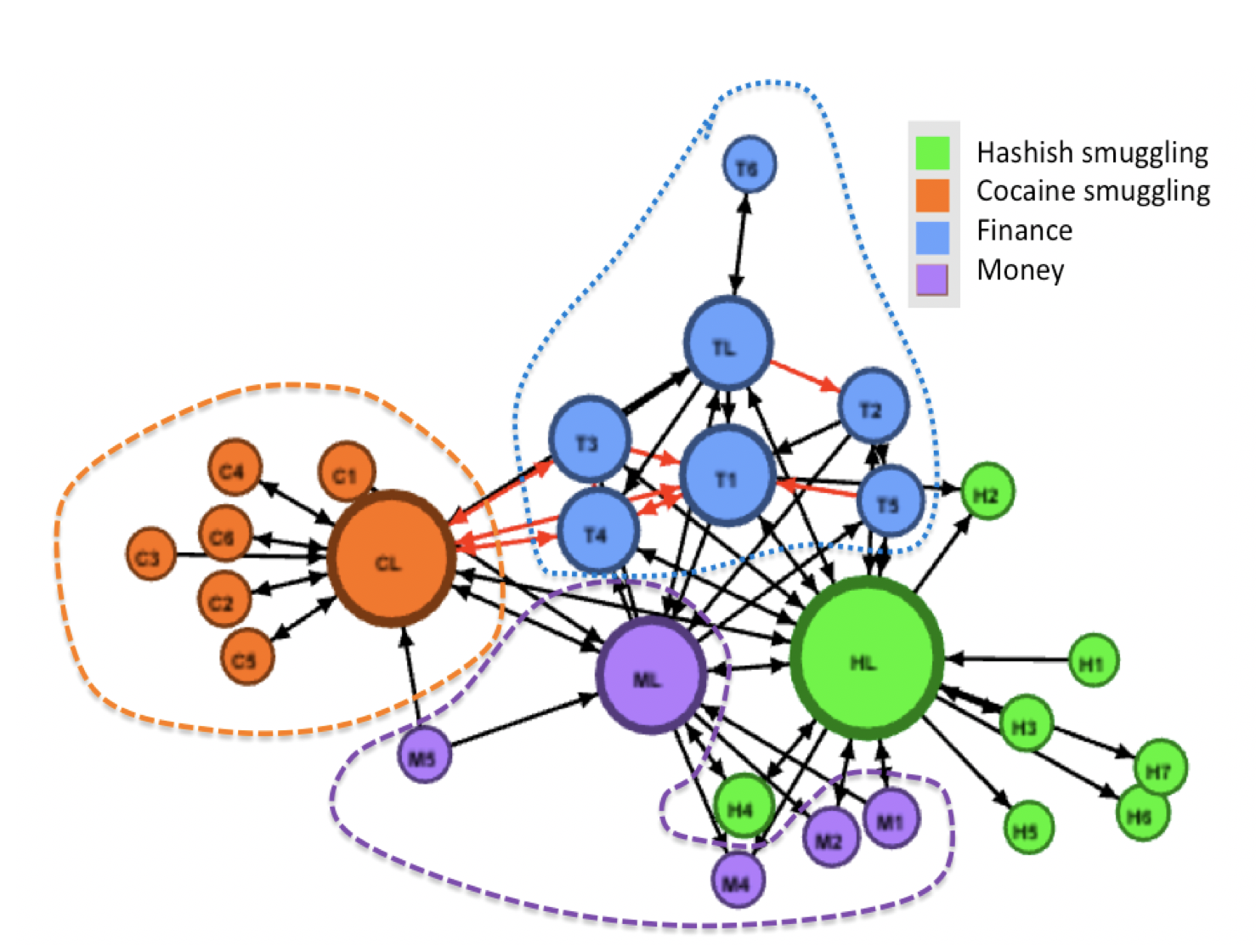
It is well-known that Transnational Crime Organizations (TCOs) actively try to obfuscate their structures and hierarchies. This creates direct challenges to designing analytical tools for recreating TCOs network structures from uncertain data, and indirectly, such tools for planning efficient interdiction strategies. This research addresses these challenges by creating prototype software that quantifies the uncertainty of a network structure created from noisy data, and creating alternative interpretations of noisy data by assigning to each alternative network structure the probability of being correct.
The project, led by a CINA-funded team at RPI, delivered novel prototypes for analytics for crime networks created from noisy data based on two novel ideas for which RPI received provisional U.S. patents. The prototyped analytic software enables analysts to create the most reliable structures of the network, compute this network uncertainty and its costs measured in uncertainty of the interdiction based on such a network. If such risk or cost is unacceptable, the tools will list the pairs of criminals for which collecting additional data about their communications will reduce the cost and uncertainty the most.
This patented technology can analyze partially-known criminal networks to reveal probable unknown criminals and determine the best strategy for interdiction. The new tools can help investigators to detect currently-unknown criminals and allocate resources to the most impactful strategies to disrupt criminal networks.
Research Products:
Publications:
A Network Generator for Covert Network Structures
Community Detection with Edge Augmentation in Criminal Networks
Measuring the impact of improved coordination in disrupting illicit trafficking networks
Dynamics of Crime Activities in the City Neighborhood Network
Interdicting Interdependent Contraband Smuggling, Money and Money Laundering Networks
Optimizing edge sets in networks to produce ground truth communities based on modularity
PhD Thesis:
Dynamics and Stability of Edges and Communities in Social Networks